The Next Step Change in Simulation Performance
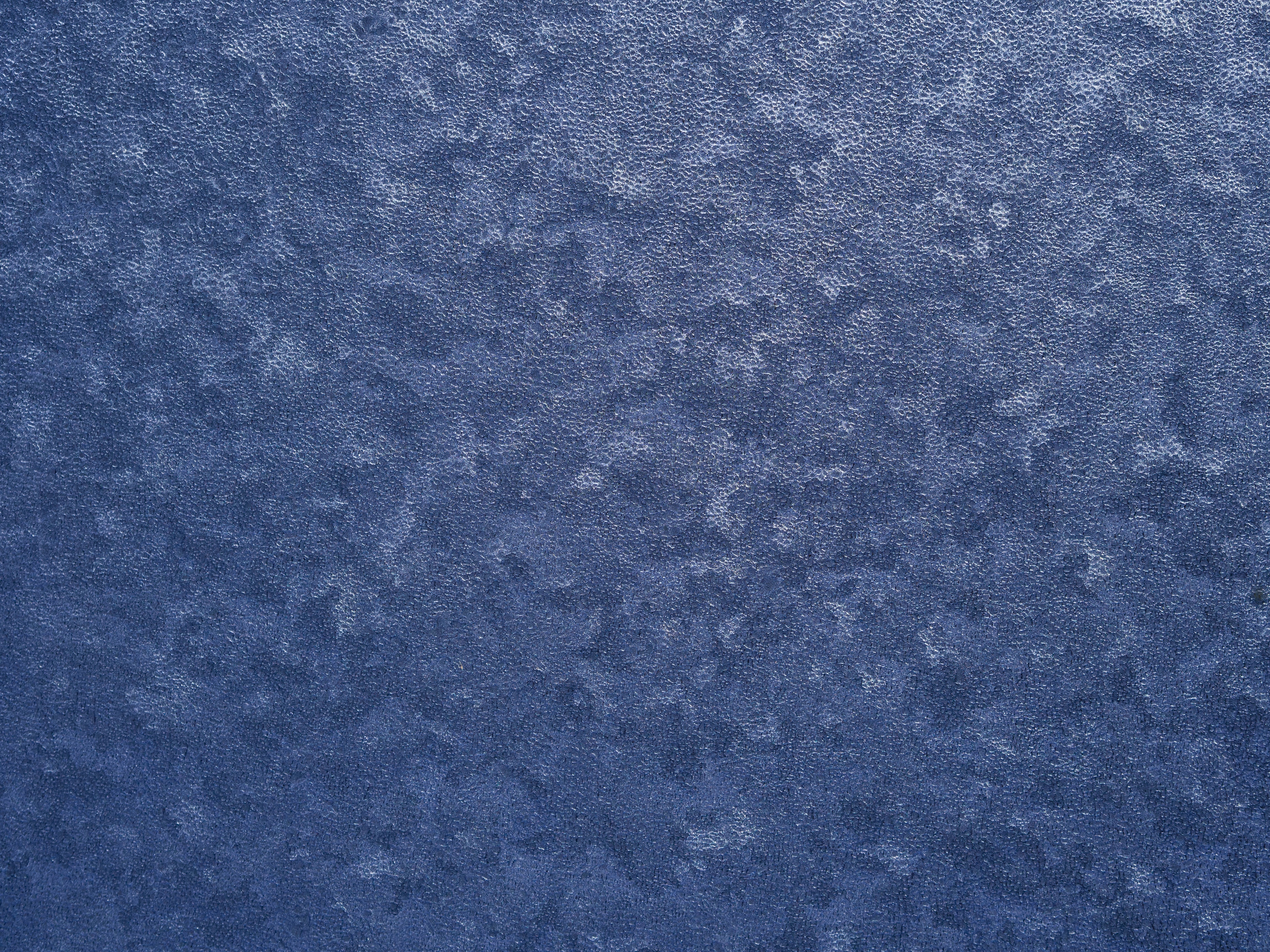
Simulation technology plays an important role in advancing innovation as an alternative to running costly experiments. Using simulators, scientists can screen drug-candidate molecules to discover new drugs, engineers can design aerofoils that minimize drag, and climate scientists can study the impact of pollution on our environment.
Although in many cases simulators provide cost-effective alternatives to real-life experiments, they are inherently associated with long compute times and may require the use of supercomputers. This challenge is compounded by the fact that most tasks require running simulators hundreds or thousands of times. In practice, the time available to explore the space of simulation parameters is limited by the project schedule and time-to-market constraints. So faster computation translates into better exploration.
One promising way to accelerate this process is to construct an intelligent system that can quickly and accurately predict the simulation output without needing to run the simulation itself. This approach is known as emulation. When done right, emulation technology can deliver similar predictions to simulators, but without the need for supercomputers.
In our peer-reviewed paper, we show how such an intelligent emulator can be realized by deploying machine learning (ML) technology. A few hundred or thousand simulation datasets are needed to construct the emulator, and once complete, the emulator can predict new results quickly, without having to run additional expensive simulations. In one case, our ML-based emulation technology predicted the output of more than 250 simulation runs in less than a second using a single GPU, versus an original cost of 1100 CPU hours per simulation run. Further, we also demonstrated that our ML emulation technology is capable of delivering results with high accuracy. We show below an example of the simulation output versus our emulator prediction in the case of oceanic chemical concentration simulations.
Our ML-based emulation technology opens up new applications that require fast calculations, of broad benefit to engineering, semiconductor design, clean energy, drug discovery, finance, online diagnostics, and research. With the substantial acceleration achieved by ML-based emulation technology, we believe our approach holds the key to delivering the next step-change in predictability, as a companion to the existing simulators.